Cold Water Prediction: Using Artificial Intelligence to Protect Sea Life During Winter Freezes
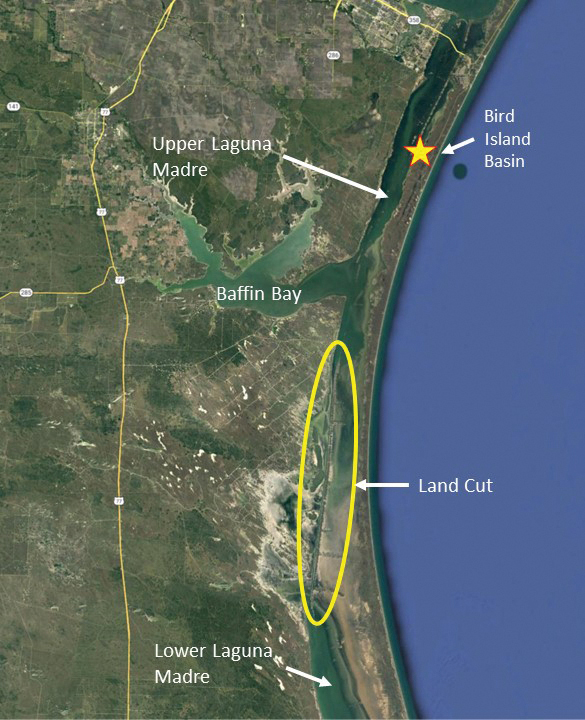
Figure 1: Map of the Laguna Madre showing the location of Bird Island Basin and the Land Cut.
Sudden and severe water temperature drops have resulted in substantial fish kills and sea turtle strandings along the Texas coast. Concerns about maritime commerce exacerbating these impacts in the Laguna Madre have been expressed as far back as the 1980s. Stakeholders were concerned fish were using the Intra Coastal Water Way (ICWW) as a thermal refuge in the Laguna Madre (LM) and barge traffic was impacting those fish. So, members of the Gulf Intracoastal Canal Association (GICA), the Coastal Conservation Association (CCA), Texas A&M-Corpus Christi (TAMU-CC), and Texas Parks and Wildlife Department (TPWD) sought to develop strategies to mitigate impacts related to barge operations and to balance impacts to natural resources with the economic impact on barge operators. One mitigation strategy is the voluntary suspension of barge operations when water temperatures are predicted to fall below a threshold of 40 Degrees Fahrenheit for over 24 hours. But the challenge was predicting the drop in water temperature early enough for barge operators to adjust operations. To do this an accurate and precise water temperature model was needed.
In 2006, the TAMU-CC Conrad Blucher Institute (CBI) began development of the water temperature predictive model. The first phase of the project installed water temperature profiling stations within the Intracoastal Waterway, in and around the “land cut”, which is the southern section of the ICWW that was dredged across an extensive tidal flat connecting the Upper and Lower Laguna Madre. Water temperature sensors were deployed at multiple depths to measure water temperatures from the surface water to the bottom sediment. By monitoring these depth profiles, the study team concluded water temperatures were similar throughout the water column making it unlikely that fish seek thermal refuge at the bottom of the Intracoastal Waterway. Researchers also concluded that water temperatures were similar throughout the LM by comparing results with other stations throughout the system, except in Baffin Bay.
The next step was to understand what conditions influence water temperature changes within the system. For example, a frontal system passes through the region and air temperature drops. Winds associated with the front mixes the water, dissipating the heat from the water column. The shallowness of the Laguna Madre causes water temperatures to drop at a faster rate than surrounding areas like Corpus Christi Bay or Baffin Bay. By using this concept, researchers sought to quantify observations and environmental responses for model development. Air temperature was identified as the main driver influencing water temperature because the of system’s shallowness. Statistical analysis also highlighted the relative importance of time lags for predicting changes in water temperature. These observations and data collected from water temperature profiling gave researchers the information needed to develop the water temperature prediction model.
Artificial Intelligence (AI) was employed for model development. Programming techniques included the use of Machine Learning methods such as random forests (RF) and Artificial Neural Networks (ANN). Machine learning uses mathematical algorithms to build a model based on samples of data, known as training data. Analysis of the training data allowed the model to “learn” how to make predictions. Training data for developing the model included water and air temperature measurements at the Bird Island Basin (BIB) Station in the northern portion of the Upper Laguna Madre and predicted air temperature from NOAA. BIB has a long time series of data, 20 years, with limited data interruptions and captured numerous cold events in the study area. The result is a model that can make predictions about water temperatures based on the data from the field. The model ran successfully for many years
In 2017, CBI and sea turtle researchers presented findings of their study highlighting cold water temperature thresholds for sea turtles. This included a proposal to increase the water temperature threshold from 40 degrees to 46 degrees Fahrenheit over a 24-hour period. GICA agreed to the proposal for expanding mitigation efforts to include fisheries and sea turtles in response to extreme cold-water events. By this time predictions were calculated manually due to aging IT software and other infrastructure issues. A model overhaul was also necessary.
In 2018 funding became available to restructure the model into a water temperature predictive system. Newer IT tools and development of a new database, software, and additional data source improved the model, its analytics, and its resiliency. The system now consists of several consolidated ANN models to predict falling water temperature and duration. A sensitivity analysis assessed the impact of imprecise air temperature predictions to improve model performance. A new database and software were developed to ingest and store NOAA predictions. Additionally, air temperature predictions were ingested from the IBM Global High-Resolution Atmospheric Forecasting System (IBM GRAF) into the system as part of collaborations within one of the inaugural National Science Foundation AI institutes, AI2ES.org. This new global weather model updates hourly and at a 3 – 4 km resolution for improved analytics. Instead of providing one air temperature prediction per location/lead time, IBM provides the team a range of predictions allowing researchers to get a better idea of how confident the models are and how likely deviations are from the main prediction.
In 2020 the automated cold water prediction system had not been completed. The initial prediction of a drop in water temperature made by researchers was accurate. However, the duration of the water temperature drop proved to be challenging and was predicted to be too short. The concerns researchers had about manual water temperature predictions came to fruition. If the automated system had been operational, the guidance ahead of Winter Storm Uri would have been better, likely proposing a recommendation for a longer navigation and coastal work interruption. Following this event, data was captured and applied to the systems for machine learning purposes.
By fall 2021, the full system was in place and put to the test in February 2022. It accurately predicted the initial drop in water temperature below 47 deg F for over 24 hours and predicted the duration of the event. The prediction assisted in mitigating the impacts of a very large cold stunning event in the LM system by prompting the issuance of a U.S. Coast Guard notice to mariners and the request by GICA for its members to modify barge operations. Also, volunteers were alerted in time and many organizations coordinated wildlife rescuers to save over 400 sea turtles.
Thanks to the 20-year collaborative development of the water temperature predictive system, modifications to barge operations and mitigation efforts have been successfully implemented along the Texas coast during cold weather events. The predictive model’s success is the result of a successful collaboration between the maritime transportation industry, state, and federal partners, as well as invested stakeholders.